Fairness in Machine Learning
Publications
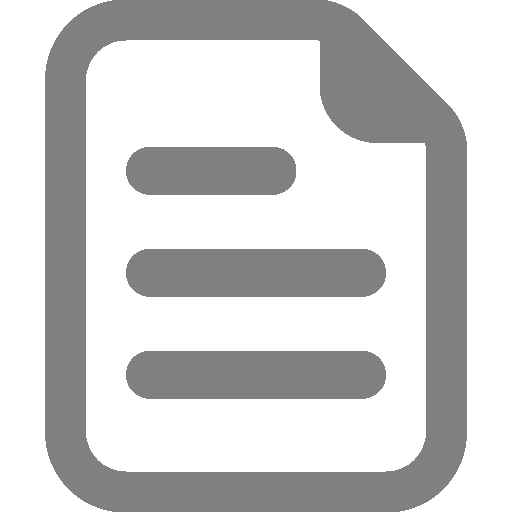
@inproceedings{romanov2019s, title={What's in a Name? Reducing Bias in Bios without Access to Protected Attributes}, author={Romanov, Alexey and De-Arteaga, Maria and Wallach, Hanna and Chayes, Jennifer and Borgs, Christian and Chouldechova, Alexandra and Geyik, Sahin and Kenthapadi, Krishnaram and Rumshisky, Anna and Kalai, Adam}, booktitle={Proceedings of the 2019 Conference of the North American Chapter of the Association for Computational Linguistics: Human Language Technologies, Volume 1 (Long and Short Papers)}, pages={4187–4195}, year={2019} }